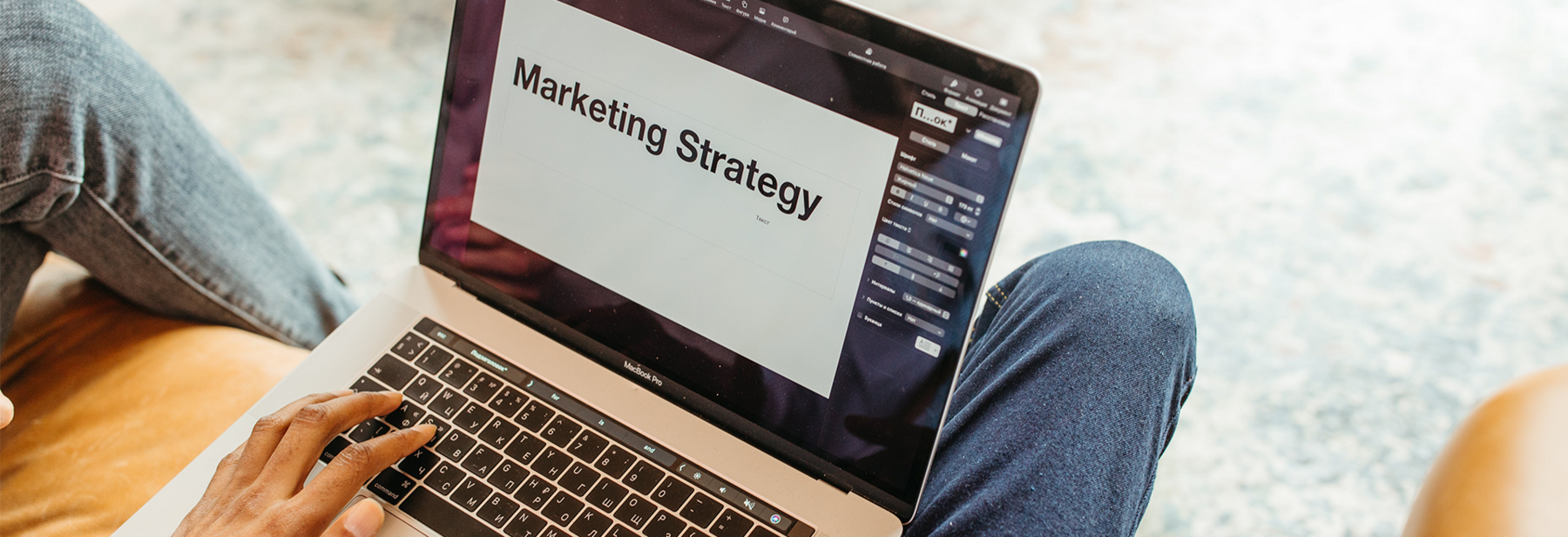
A New Hierarchical Bayes Geographically Weighted Regression Spatial Model for Customer Service and Satisfaction Measurement In Marketing Research
Sunghoon Kim, Arizona State University
We propose a new Hierarchical Bayes Geographically Weighted Regression model which helps marketing researchers to calibrate the potential impact of spatial dependency and heterogeneity on the underlying drivers of customer service and/or satisfaction measurement. This proposed procedure accommodates a hierarchical structure and non-stationary geographical boundary effects. Using several synthetic datasets, we first illustrate the proposed model outperforming three benchmark competing models including ordinary linear regression, classic Geographically Weighted Regression, and Bayesian Geographically Weighted Regression, especially when there exist significant state-level fixed effects or irregular patterns of observation locations. In the automobile customer satisfaction application study provided, the proposed procedure also demonstrates favorable performance in predictive validation measures compared to these same benchmark models. Here, we also derive a clear heterogeneous pattern of two covariates in the Mountain U.S. geographic division examined: dealership service is highest in the northeastern Mountain regions (e.g., Montana), and vehicle quality is highest in the southern Mountain regions (e.g., Arizona, New Mexico).